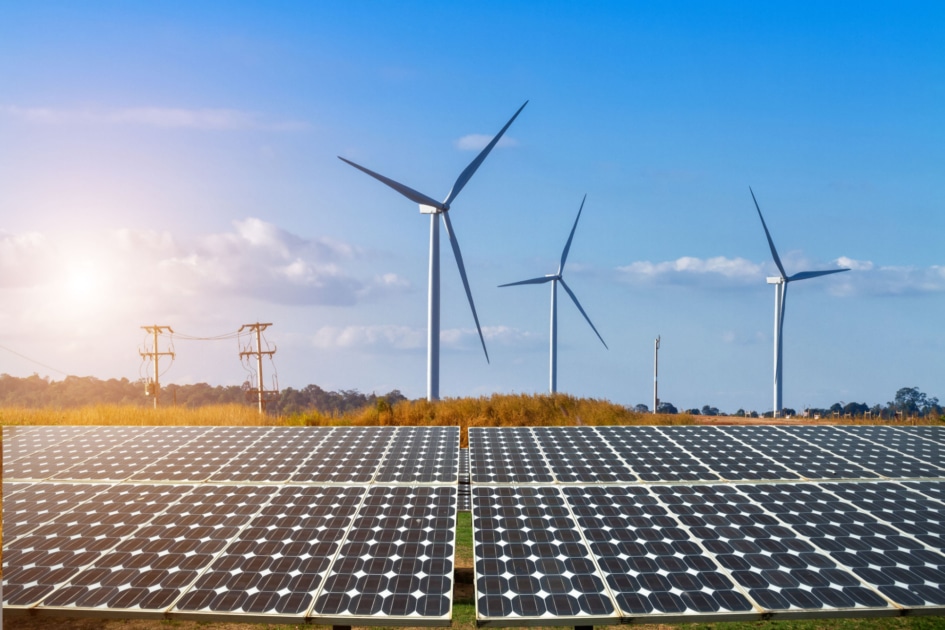
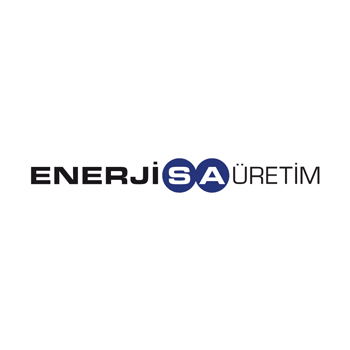
About Enerjisa Üretim
Enerjisa Üretim was established in 1996 in Istanbul to champion a sustainable balance in power generation. The company has a diversified and efficient portfolio and is now the market leader in Turkey’s private sector electricity generation. With 850 employees the company has 21 power plants, a 3.607MW installed capacity, and 56% of its power generation comes from renewable energy.
“At Enerjisa Üretim, our new self-service data analytics tool has enabled us to go from data visualization to data interrogation across multiple systems and sources. Now plant engineers can set alarms triggered by efficiency tags. This is driving our corporate strategy to achieve operational excellence and meet sustainability targets.”
Emin SahinPower Plants Performance Monitoring and Development Mentor, Enerjisa Üretim
Their Digitalization Journey & Business Challenges
Enerjisa Üretim has 3 wind power plants, 12 hydroelectric power plants, 2 solar power plants, 3 natural gas power plants and 1 lignite power plant. The ongoing devaluation of the lira makes its provision of affordable clean energy an essential part of its business plan. And like any other power plant, better asset management is key to operational efficiency and cost savings.
In its hydroelectric power plant, Enerjisa has huge turbines responsible for producing electricity. While in its natural gas plant, coolers are vital to condense the steam. Data around both these processes are critical to ensuring that the asset operates efficiently. Enerjisa knows this, which is why it is using a web-client visualization tool (PI) to show time-series data in dashboards. This used to help with simple diagnostics.
But plant managers were starting to get frustrated: there were several irregularities in equipment performance or operating anomalies causing latency or downtime without anyone really knowing why. But management didn’t think they could make their data work any better for them.
Solution & Implementation
With TrendMiner they were able to conduct powerful analyses of combined data sources from remote monitoring, internal systems, and time-series data from the past 3 years. In just one week, Enerjisa operators (not data scientists) had established 4 groundbreaking use cases for optimizing operations.
Use Case 1: Correlation analysis of cooler
Goal: To analyze the influence of meteorological parameters on cooler performance.
Results: Using scatter plots, operators found a strong negative correlation (-80%) between the ambient temperature and the cooler’s power output. They identified 12 cases over the past year where the cooler had not even kicked in despite extreme weather conditions.
Use Case 2: Correlation analysis of steam turbine
Goal: As above but with steam turbines.
Results: Contrary to previous belief operators established that atmospheric pressure did not influence the process as much as humidity does.
Use Case 3: Optimize pump operations
Goal: To understand operational impact of pump failure.
Results: The team compared periods where all pumps were operating to periods when one wasn’t. Now, they have alerts to ensure that pump downtime is avoided.
Use Case 4: Axial shaft position
Goal: To analyze the evolution of the axial shaft position (slowly displaced over time) and predict trips based on this displacement.
Results: Established a predictive maintenance schedule.
Benefits
- TrendMiner enabled three Enerjisa Üretim plants to achieve operational efficiency through remote monitoring, and predictive maintenance. The advanced analytics have made it possible to:
- Eliminate data silos
- Achieve 4 compelling use cases in under a week
- Establish negative correlation (-80%) between the temperature and power output
- Detect 12 unidentified failures
- Perform fast root cause analysis on complex data sets
- Offer flexible price-on-volume data/users model
Engineers will soon start using TrendMiner’s Notebooks functionality. This new intelligence layer sees all that complex operational data fed and analyzed by Machine Learning. Using Python, it’s possible for developers to program and to create new smarter dashboards for operators to interact with.
About Enerjisa Üretim
Enerjisa Üretim was established in 1996 in Istanbul to champion a sustainable balance in power generation. The company has a diversified and efficient portfolio and is now the market leader in Turkey’s private sector electricity generation. With 850 employees the company has 21 power plants, a 3.607MW installed capacity, and 56% of its power generation comes from renewable energy.
“At Enerjisa Üretim, our new self-service data analytics tool has enabled us to go from data visualization to data interrogation across multiple systems and sources. Now plant engineers can set alarms triggered by efficiency tags. This is driving our corporate strategy to achieve operational excellence and meet sustainability targets.”
Emin SahinPower Plants Performance Monitoring and Development Mentor, Enerjisa Üretim
Their Digitalization Journey & Business Challenges
Enerjisa Üretim has 3 wind power plants, 12 hydroelectric power plants, 2 solar power plants, 3 natural gas power plants and 1 lignite power plant. The ongoing devaluation of the lira makes its provision of affordable clean energy an essential part of its business plan. And like any other power plant, better asset management is key to operational efficiency and cost savings.
In its hydroelectric power plant, Enerjisa has huge turbines responsible for producing electricity. While in its natural gas plant, coolers are vital to condense the steam. Data around both these processes are critical to ensuring that the asset operates efficiently. Enerjisa knows this, which is why it is using a web-client visualization tool (PI) to show time-series data in dashboards. This used to help with simple diagnostics.
But plant managers were starting to get frustrated: there were several irregularities in equipment performance or operating anomalies causing latency or downtime without anyone really knowing why. But management didn’t think they could make their data work any better for them.
Solution & Implementation
With TrendMiner they were able to conduct powerful analyses of combined data sources from remote monitoring, internal systems, and time-series data from the past 3 years. In just one week, Enerjisa operators (not data scientists) had established 4 groundbreaking use cases for optimizing operations.
Use Case 1: Correlation analysis of cooler
Goal: To analyze the influence of meteorological parameters on cooler performance.
Results: Using scatter plots, operators found a strong negative correlation (-80%) between the ambient temperature and the cooler’s power output. They identified 12 cases over the past year where the cooler had not even kicked in despite extreme weather conditions.
Use Case 2: Correlation analysis of steam turbine
Goal: As above but with steam turbines.
Results: Contrary to previous belief operators established that atmospheric pressure did not influence the process as much as humidity does.
Use Case 3: Optimize pump operations
Goal: To understand operational impact of pump failure.
Results: The team compared periods where all pumps were operating to periods when one wasn’t. Now, they have alerts to ensure that pump downtime is avoided.
Use Case 4: Axial shaft position
Goal: To analyze the evolution of the axial shaft position (slowly displaced over time) and predict trips based on this displacement.
Results: Established a predictive maintenance schedule.
Benefits
- TrendMiner enabled three Enerjisa Üretim plants to achieve operational efficiency through remote monitoring, and predictive maintenance. The advanced analytics have made it possible to:
- Eliminate data silos
- Achieve 4 compelling use cases in under a week
- Establish negative correlation (-80%) between the temperature and power output
- Detect 12 unidentified failures
- Perform fast root cause analysis on complex data sets
- Offer flexible price-on-volume data/users model
Engineers will soon start using TrendMiner’s Notebooks functionality. This new intelligence layer sees all that complex operational data fed and analyzed by Machine Learning. Using Python, it’s possible for developers to program and to create new smarter dashboards for operators to interact with.
Subscribe to our newsletter
Stay up to date with our latest news and updates.
Other Webinars on demand
Explore Our Newest Content to Maximize Your Operational Efficiency
Other Resources
Explore Our Newest Content to Maximize Your Operational Efficiency