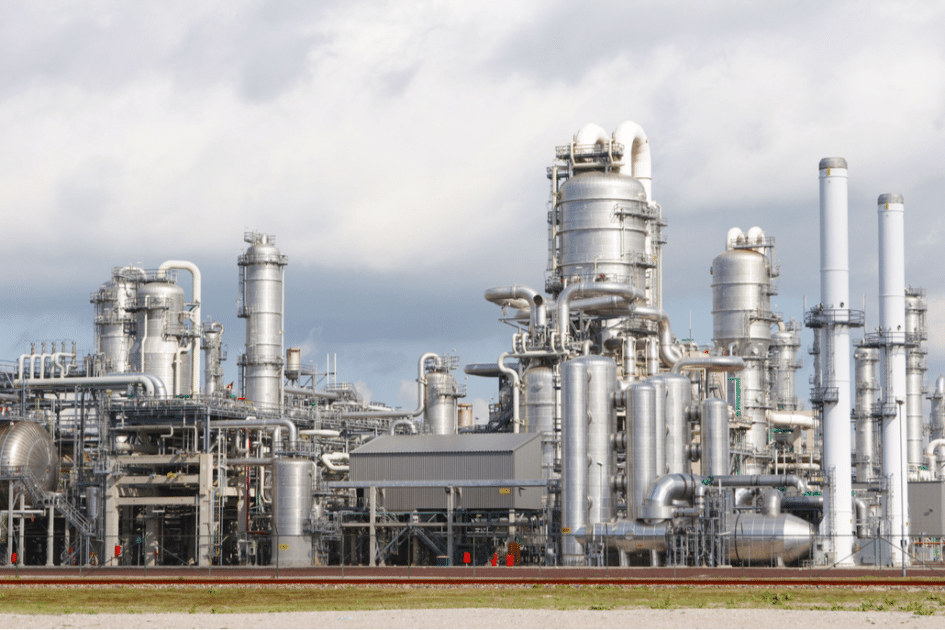
Situation
The team at the chemical plant is responsible for purifying the product by batch crystallization. The only critical component for the product specification is the remaining concentration of the stabilizer. To meet the product specification, the crystallization is performed in a falling film crystallizer in multiple, consecutive cycles. It is desirable to minimize the cycle time in order to increase the throughput. Consistently short cycles further allow a predictable production scheduling.
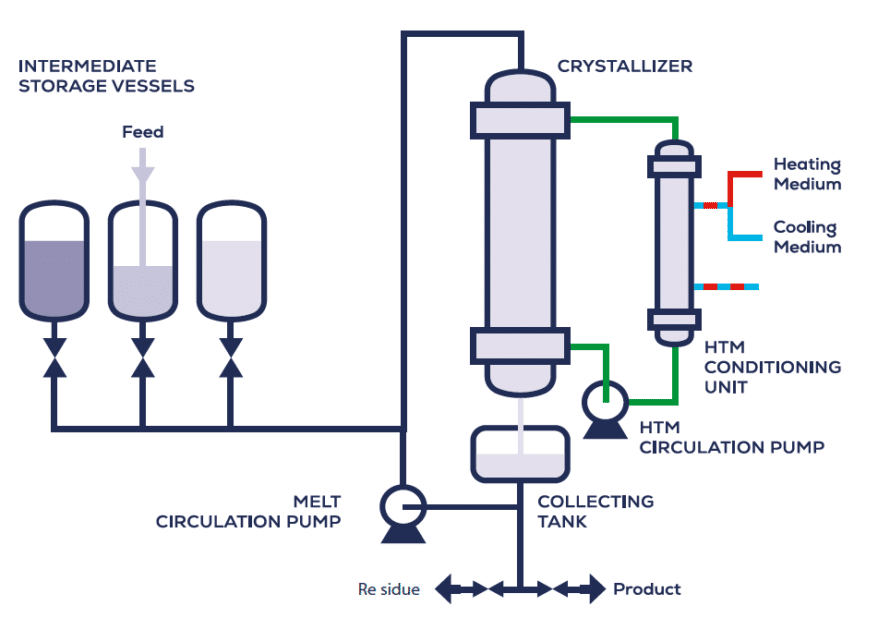
Problem
Since the last turnaround, sporadic off-spec batches were observed. This was caused by incomplete melting in the final cycle of the previous batch, which could not be explained. As a result, the concentration of the stabilizer was above specification, and a higher mass needed to be crystallized in the following batch which extended the required batch time.
Solution
The engineers already tried troubleshooting the issue, but that was not possible with their existing data analysis tooling (PIMS, Historian, Excel). These tools did not enable the engineers to compare the current run with best runs (“Golden batch fingerprint”). So the goal was to use TrendMiner and perform a root cause analysis of incomplete melting cycles to achieve consistency in batch profiles and cycle times.
1. Impact Analysis
- Searched for incomplete melting cycles using the Similarity Search.
- Layered multiple profiles and compared them against each other.
2. Compare Cycle Time
- Compared the set of incomplete cycles against a set of complete cycles (“golden batch fingerprint”).
- Checked the profiles for deviations which indicated that the cycle
was stopped too early.
3. Monitor Performance
- Used the golden batch fingerprint to visually follow up the current batches and automatically monitor for fingerprint deviations.
- Set up alerts for these deviations which also included sending email notifications to process experts.
Results
Deviations from the ideal behavior were easily identified. A consistently earlier decrease of the level (draining) in the crystallizer indicated that the melting cycle ended too early. Hence, the achieved melting temperature was insufficient for complete melting.
- Draining the liquid of the crystallizer should have been triggered by a certain value of the melting temperature in the DCS. This criterion was consistently not met for all incomplete melting cycles.
- Reconfiguration of DCS resulted in complete melting cycles.
Situation
The team at the chemical plant is responsible for purifying the product by batch crystallization. The only critical component for the product specification is the remaining concentration of the stabilizer. To meet the product specification, the crystallization is performed in a falling film crystallizer in multiple, consecutive cycles. It is desirable to minimize the cycle time in order to increase the throughput. Consistently short cycles further allow a predictable production scheduling.
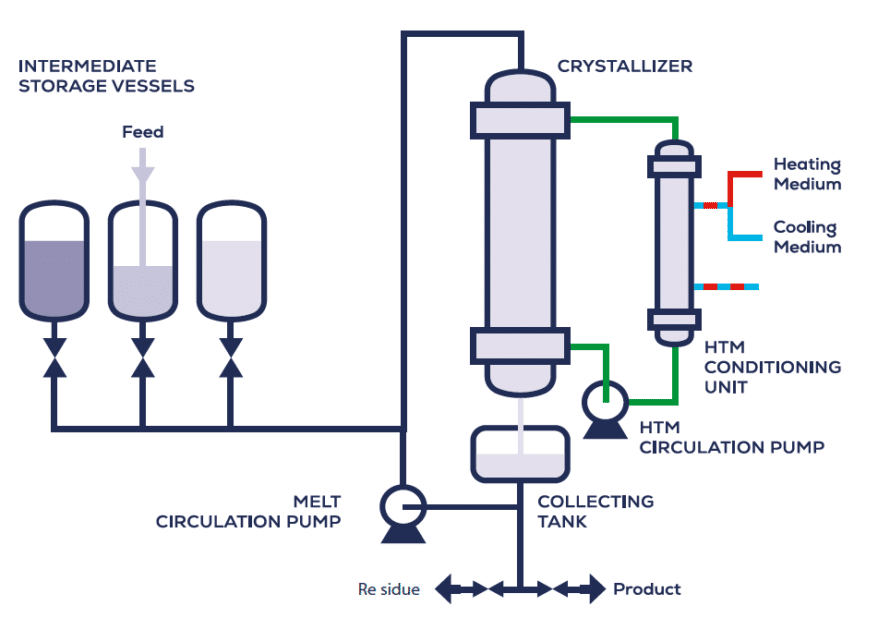
Problem
Since the last turnaround, sporadic off-spec batches were observed. This was caused by incomplete melting in the final cycle of the previous batch, which could not be explained. As a result, the concentration of the stabilizer was above specification, and a higher mass needed to be crystallized in the following batch which extended the required batch time.
Solution
The engineers already tried troubleshooting the issue, but that was not possible with their existing data analysis tooling (PIMS, Historian, Excel). These tools did not enable the engineers to compare the current run with best runs (“Golden batch fingerprint”). So the goal was to use TrendMiner and perform a root cause analysis of incomplete melting cycles to achieve consistency in batch profiles and cycle times.
1. Impact Analysis
- Searched for incomplete melting cycles using the Similarity Search.
- Layered multiple profiles and compared them against each other.
2. Compare Cycle Time
- Compared the set of incomplete cycles against a set of complete cycles (“golden batch fingerprint”).
- Checked the profiles for deviations which indicated that the cycle
was stopped too early.
3. Monitor Performance
- Used the golden batch fingerprint to visually follow up the current batches and automatically monitor for fingerprint deviations.
- Set up alerts for these deviations which also included sending email notifications to process experts.
Results
Deviations from the ideal behavior were easily identified. A consistently earlier decrease of the level (draining) in the crystallizer indicated that the melting cycle ended too early. Hence, the achieved melting temperature was insufficient for complete melting.
- Draining the liquid of the crystallizer should have been triggered by a certain value of the melting temperature in the DCS. This criterion was consistently not met for all incomplete melting cycles.
- Reconfiguration of DCS resulted in complete melting cycles.
Subscribe to our newsletter
Stay up to date with our latest news and updates.
Other Webinars on demand
Explore Our Newest Content to Maximize Your Operational Efficiency
Other Resources
Explore Our Newest Content to Maximize Your Operational Efficiency